Faculties and Schools
The University of Waikato features a structure of academic, research, and service departments, with its faculties and schools arranged within specialised divisions.
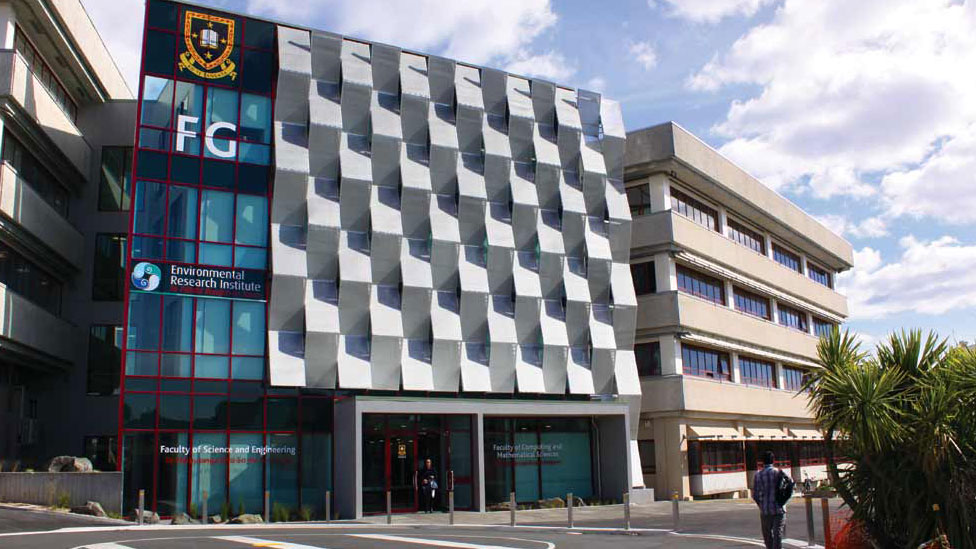
-
Te Kura Toi | School of Arts
-
Au Reikura | School of Computing and Mathematical Sciences
-
Te Kura Toi Tangata | School of Education
-
Te Kura Mata-Ao | School of Engineering
-
Te Mata Kairangi | School of Graduate Research
-
Division of Health
-
Te Piringa | Faculty of Law
-
Te Pua Wānanga ki te Ao | Faculty of Māori and Indigenous Studies
-
Te Kura Whatu Oho Mauri | School of Psychological and Social Sciences
-
Te Aka Mātuatua | School of Science
-
Te Raupapa | Waikato Management School
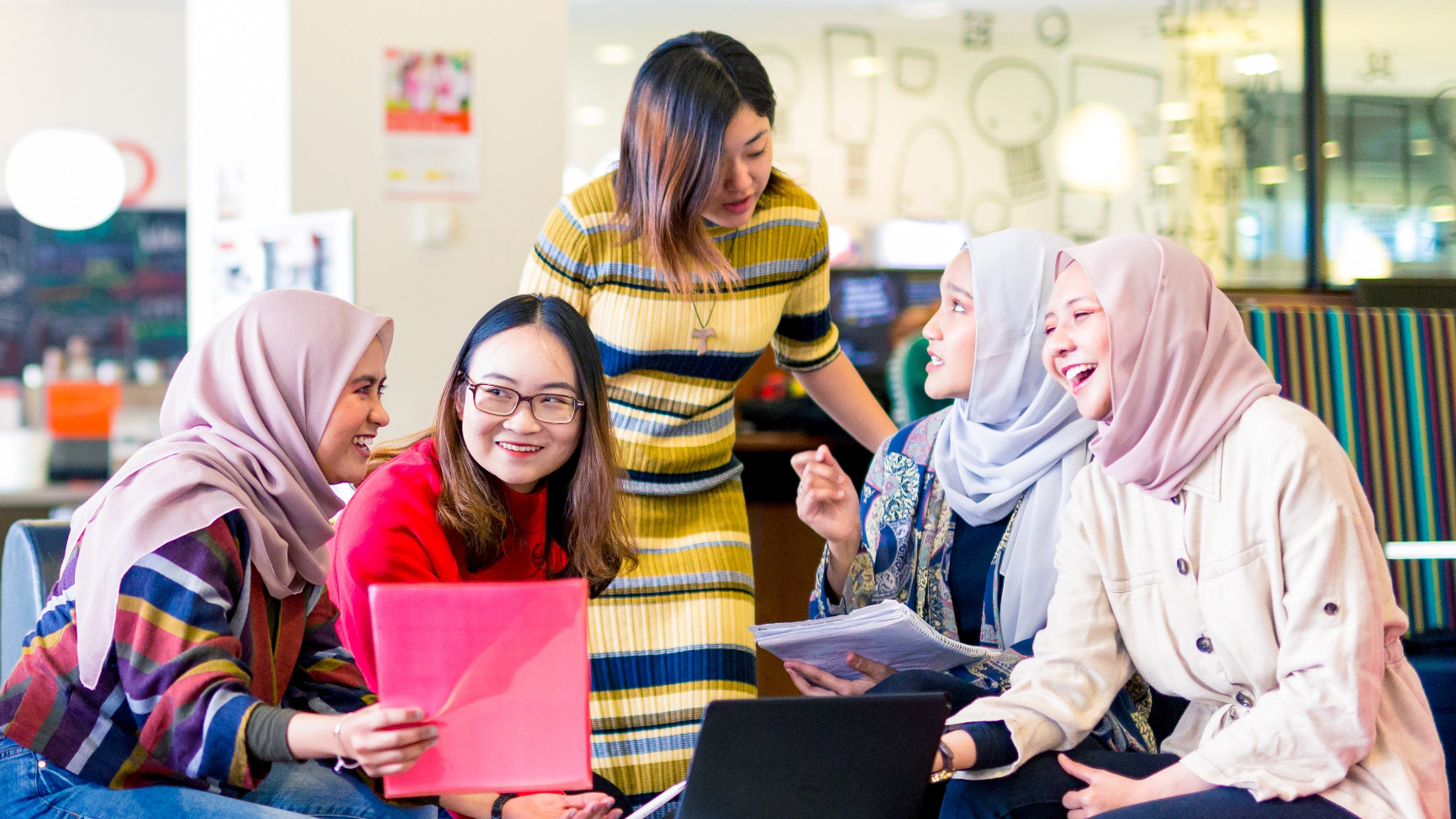
Te Kura Huanui | University of Waikato College
The College offers university preparation courses, and foundation, bridging and English language programmes. They also support international study groups in range of discipline areas and train teachers who want to teach English to adults.